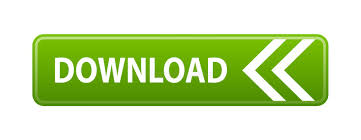
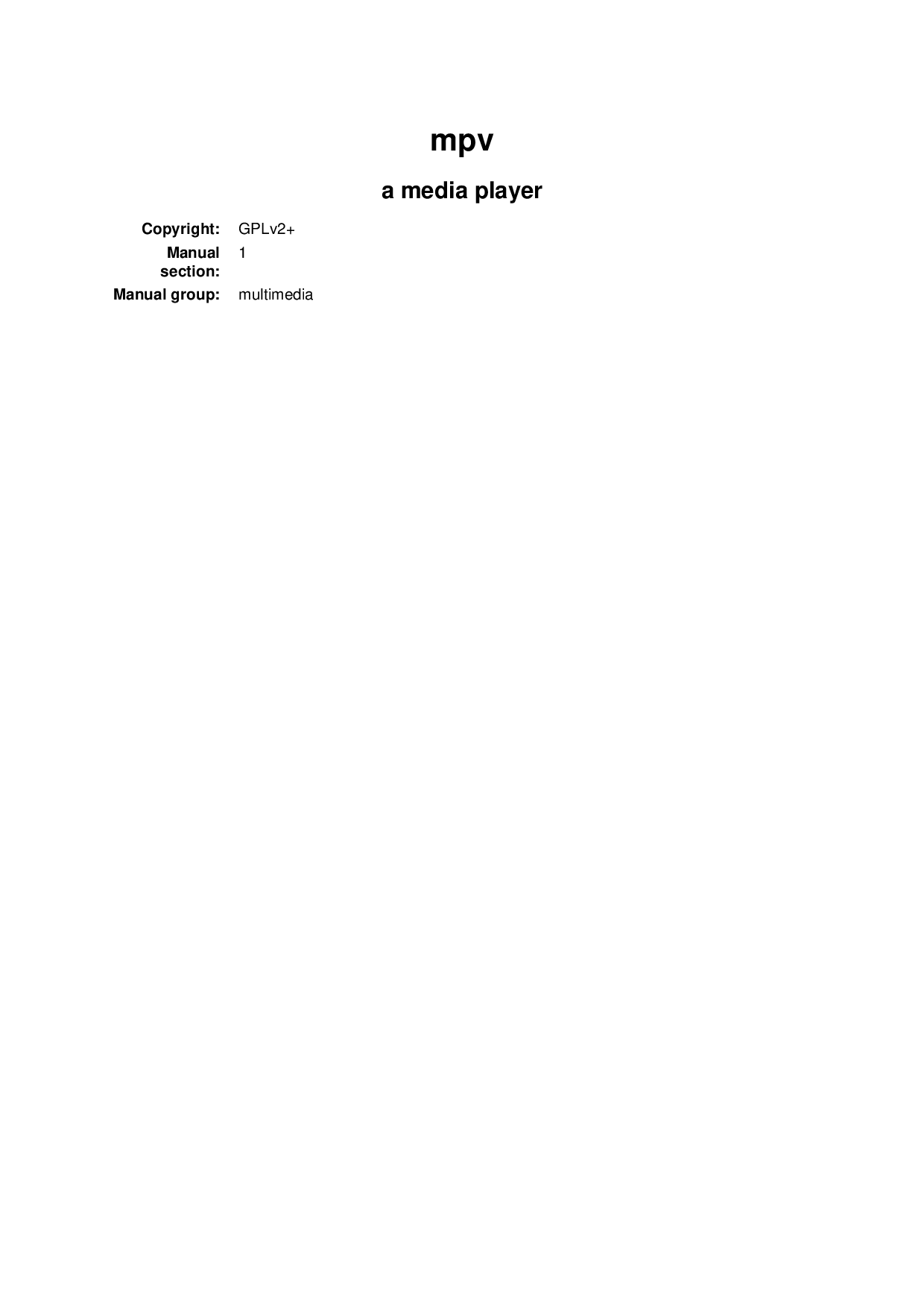
RESULTS: Our proposed architecture outperforms state-of-the-art architectures for multi-class cancer classification of HCC histopathology images, not just in terms of quality of classification, but also in computational efficiency on the novel proposed KMC liver data and the publicly available TCGA-LIHC dataset. To prove the robustness and efficacy of our models, we have shown results for two liver histopathology datasets-a novel KMC dataset and the TCGA dataset. We classify liver histopathology data into four classes, namely the non-cancerous class, low sub-type liver HCC tumor, medium sub-type liver HCC tumor, and high sub-type liver HCC tumor. As part of our experiments with this framework, we have studied the addition of atrous spatial pyramid pooling (ASPP) blocks to effectively capture multi-scale features in H&E stained liver histopathology data. shows great promise in using convolutional block attention modules (CBAM) for effective cancer classification in H&E stained breast histopathology images. METHOD: The BreastNet architecture proposed by Togacar et al. In this paper, we propose a novel deep learning framework to perform multi-class cancer classification of liver hepatocellular carcinoma (HCC) tumor histopathology images which shows improvements in inference speed and classification quality over other competitive methods.

Ez dicom cd viewer runtime error 48 manual#
Due to its laborious nature, we focus on alternate deep learning methods for automatic diagnosis, providing significant advantages over manual methods. A common method for liver cancer diagnosis is the manual examination of histopathology images. PURPOSE: Liver cancer is one of the most common types of cancers in Asia with a high mortality rate.
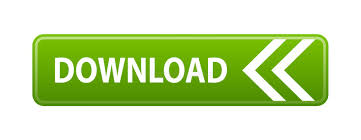